Top 5 AIOps Use Cases Shaping IT Operations in 2025
Written by
Nabia Hassan Sabzwari
Last Updated: April 18, 2025
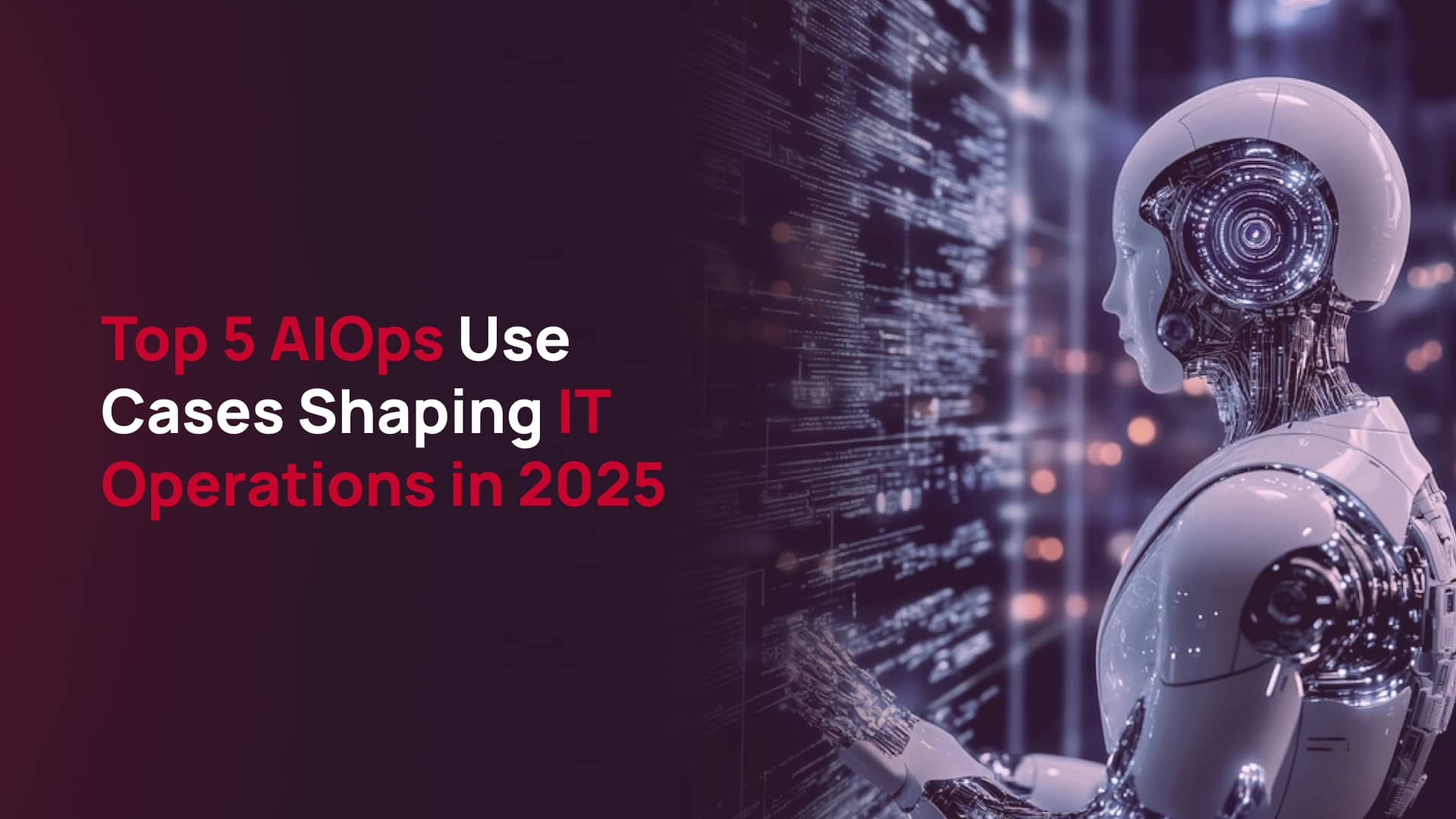
The Future of AI Starts Here
Opt for our AI development services to create intelligent, scalable and efficient solutions. Let’s push the boundaries together. Get Started with a Free Consultation Now.
AIOps is gaining significant momentum as a way to automate and optimize modern IT operations. At its core, AIOps represents the convergence of big data and machine learning to handle IT operations tasks, such as event correlation, anomaly detection and causality analysis, at scale.
As IT infrastructures grow increasingly complex, artificial intelligence for IT operations (AIOps) plays a vital role in ensuring stability, reliability, and agility. In 2025, five high-impact AIOps use cases are shaping the way IT teams manage systems, mitigate incidents and scale infrastructure.
With smarter automation and more advanced AI models, AIOps platforms and AI development companies are evolving rapidly to meet these shifting demands. These developments support the dynamic needs of today's IT environments to enable faster resolution, greater efficiency and a more proactive approach to infrastructure management.
1. Proactive detection and prevention of incidents
Among the most critical AIOps use cases in 2025 is the proactive detection and prevention of incidents. Traditional monitoring tools often fail to detect subtle anomalies that signal deeper, systemic issues, especially across distributed, hybrid environments.
Modern platforms excel at this challenge. By ingesting real-time data from logs, metrics and events across multiple systems, they offer a unified view of the entire IT environment. These platforms continuously analyze this data with machine learning to detect anomalies well before they escalate into full-blown outages.
For example, an AIOps platform may detect an unusual spike in latency on a specific API, signaling a potential failure. It can recommend preventative actions, such as scaling compute resources or reviewing the latest deployment. This kind of insight is invaluable for sectors like finance or healthcare, where downtime can mean significant financial and reputational losses.
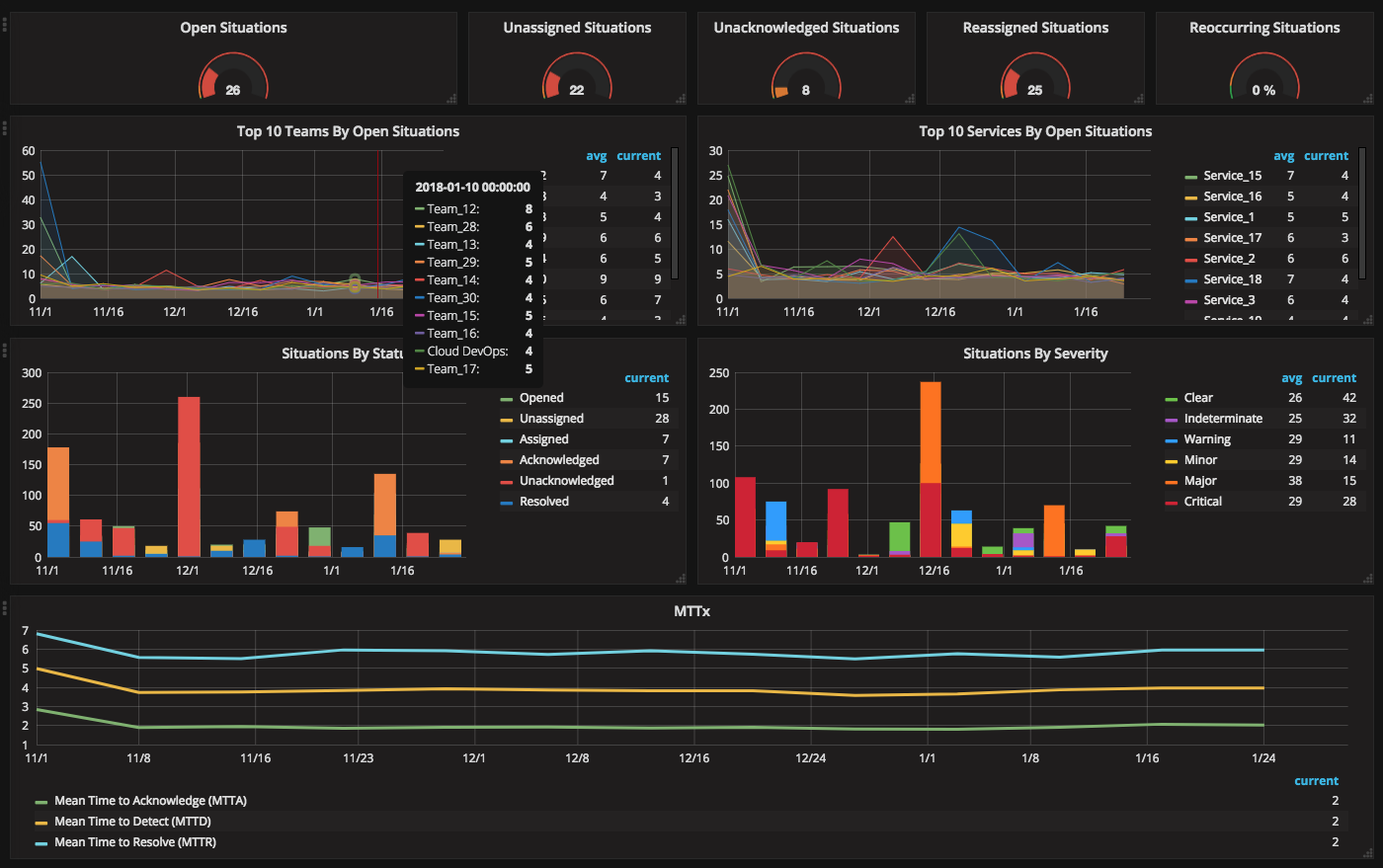
Source: Moogsoft
Unlike reactive approaches, AIOps takes a proactive stance that forms the foundation of modern IT resilience. Companies like Moogsoft and Aisera are already implementing these predictive AIOps solutions.
2. Intelligent alert noise reduction
One of the most practical and immediately beneficial AIOps use cases is intelligent alert noise reduction. As IT environments become more distributed and complex, traditional systems tend to flood teams with alerts, many of which are low-priority, redundant, or false positives.
This flood of noise creates alert fatigue, where critical signals are buried under irrelevant data. AIOps platforms solve this by using machine learning to correlate and contextualize alerts. Instead of generating dozens of separate alerts for related symptoms, AIOps groups them into a single, meaningful incident.
For instance, if a microservice fails and causes CPU spikes, API errors and latency issues, an AIOps tool like BigPanda correlates these alerts and notifies the team of one actionable problem. The result? Sharper focus, faster response times, and reduced mean time to resolution (MTTR).
This kind of intelligent triage is essential in large enterprise environments where every minute of downtime has cost implications. By filtering out the noise and surfacing only what's critical, AIOps solutions make sure that engineering teams spend their time solving problems, not chasing false alarms.
3. Automated root cause analysis
In modern IT ecosystems, identifying the source of an issue can be a massive time sink. Systems generate massive amounts of data, and tracking the origin of an outage or performance degradation often takes hours, if not days.
That’s where automated root cause analysis (RCA) becomes a game-changer. AIOps solutions can analyze massive datasets in real time, including logs, metrics, traces and event streams, to pinpoint the underlying cause of a problem with precision.
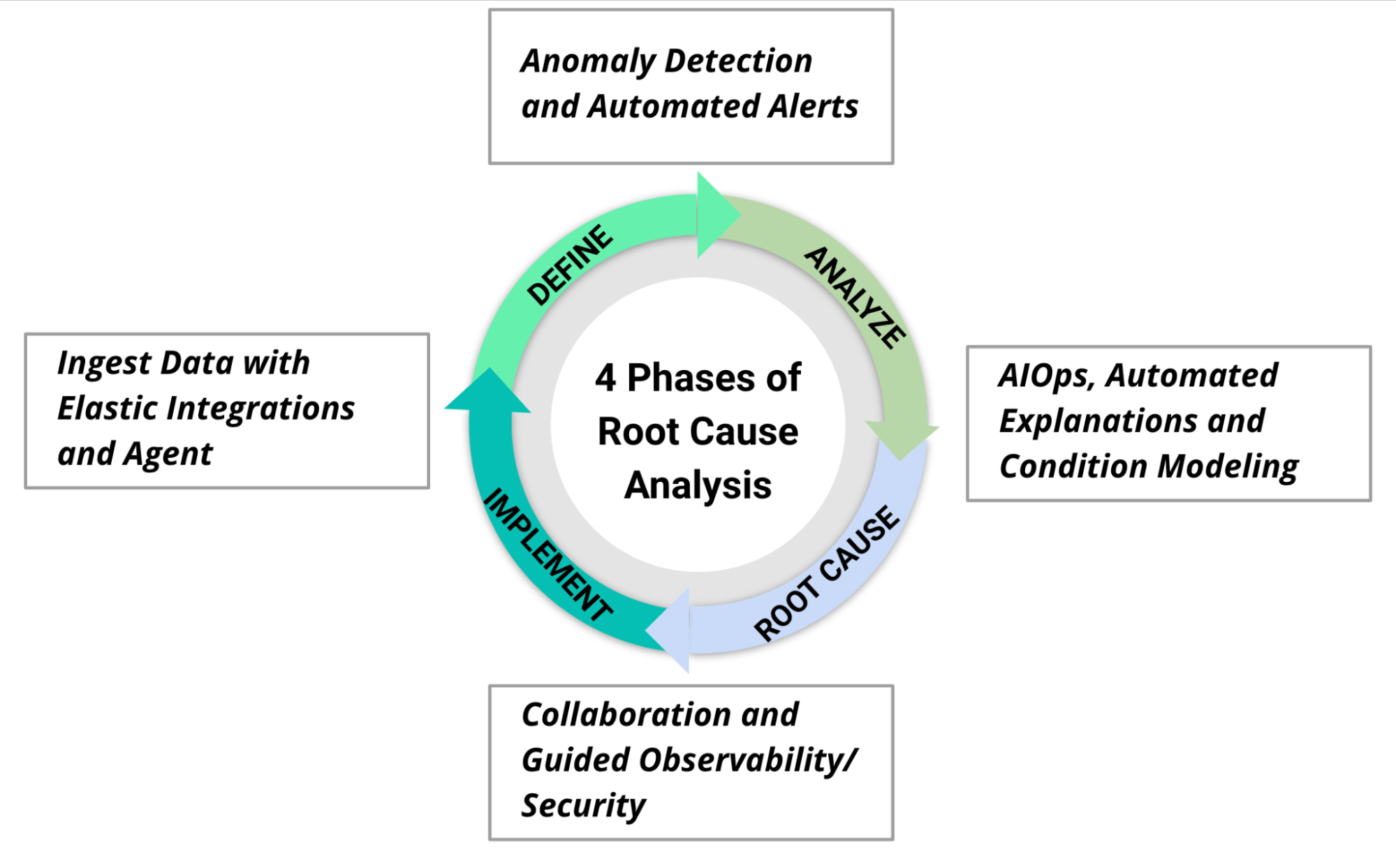
Source: Elastic.co
Say an application is running slow. A strong AIOps solution like Dynatrace can instantly trace the issue to a failed database query or an overloaded API endpoint. Instead of manually digging through logs, IT teams receive instant, actionable insight, dramatically speeding up resolution.
Behind the scenes, many organizations turn to specialized AI development services, external partners who design and deploy custom machine learning solutions, to improve the accuracy of root cause analysis. These services help tailor models to match a company's unique architecture, whether it involves microservices, legacy systems or hybrid environments, resulting in faster insights and better incident response.
As infrastructures become more interconnected, automated root cause analysis isn’t just useful, it’s essential. It reduces guesswork, lowers downtime and provides the clarity teams need to keep systems running smoothly.
4. Resource optimization & cost efficiency
Another major AIOps use case is resource optimization, a priority in cloud-native environments where workloads and costs can fluctuate unpredictably.
AIOps platforms help organizations scale infrastructure intelligently by predicting demand using historical and real-time data. This allows systems to automatically adjust compute, storage, and network resources, matching them with actual business needs.
Consider a SaaS application that experiences high traffic during work hours and lower traffic at night. AIOps can identify this pattern and automatically scale resources up or down, preventing both performance issues and unnecessary spend.
Platforms like Moogsoft and Dynatrace leverage machine learning to optimize resource allocation across environments, ensuring systems aren’t under-provisioned (causing lags) or over-provisioned (wasting budget).
This use case is especially vital for enterprises seeking sustainable growth while maintaining reliability. With no need for manual tweaking or overprovisioning, AIOps ensures your infrastructure operates efficiently and cost-effectively around the clock.
5. Generative AI for IT knowledge management & automation
The integration of generative AI into AIOps platforms is transforming the way IT teams interact with data, solve issues and manage knowledge.
Generative tools now function as intelligent copilots by automating everything from script generation and documentation to incident summaries and log interpretation.
Take the example of a cryptic error log. Instead of having engineers dig through documentation, a generative AI system can translate the log into plain language, suggest possible causes, and even generate a script to resolve the issue.
When paired with AI development services, these tools can be fine-tuned using an organization's internal data, such as configurations, runbooks and incident history, to deliver ultra-relevant results. Solutions like Aisera are already integrating generative AI to power live remediation, build knowledge base articles and automate ticket resolution.
This leads to faster response times and a continuously improving IT knowledge ecosystem. Generative AI not only helps teams operate more efficiently, but also turns every incident into a training moment for future automation.
As the technology matures, expect generative AI to become a cornerstone of AIOps strategies, augmenting human expertise and transforming how IT operations work at every level.
What are the four key stages of AIOps?
To understand how these use cases fit into broader AIOps workflows, it’s helpful to break down AIOps into its four foundational stages:
1. Monitoring
This stage involves continuously gathering data from diverse sources like servers, networks, applications, containers, cloud environments, and more. Metrics, logs, events and traces are all captured in real time to create a holistic view of the IT ecosystem.
2. Detection
AI/ML models process the incoming data to identify anomalies, performance degradation or unusual behavior. Instead of relying solely on static thresholds, these models adapt to patterns over time, improving detection accuracy and minimizing false positives.
3. Analysis
Once an issue is detected, AIOps platforms correlate signals across systems to uncover context and pinpoint root causes. This includes tracing dependencies between services, identifying cascading failures, and surfacing insights that would be hard to detect manually.
4. Remediation
In the final stage, the system takes action, either automatically or with human input. This could mean restarting a service, reallocating resources or opening a ticket with a recommended fix. The goal is to resolve problems quickly and prevent similar issues from reoccurring.
These four stages form the operational backbone of modern AIOps solutions, enabling teams to move from reactive firefighting to proactive, intelligent automation.
Conclusion
In 2025, AIOps use cases like proactive detection, alert noise reduction, automated root cause analysis, resource optimization and generative AI integration are redefining how IT operations function.
Gone are the days when businesses could afford to rely on reactive models. AIOps platforms now offer intelligent, real-time automation that helps IT teams stay ahead of incidents, optimize resources, and maintain performance at scale.
As AIOps solutions become smarter and more adaptable, AI development services will play a crucial role in helping organizations customize these systems to their unique needs, whether through tailored AI models, generative pipelines or intelligent automation workflows.
FAQs
1. What are some examples of AIOps use cases? Some common AIOps use cases include proactive incident detection, intelligent alert noise reduction, automated root cause analysis, resource optimization in cloud environments, and generative AI for IT knowledge management. These use cases help IT teams respond faster, reduce downtime, and operate more efficiently.